May 3, 2021
Matthews among star forwards testing limits of expected goal models
At some point you run out of things to say about the season Toronto Maple Leafs star Auston Matthews is having. With 38 goals in 47 games, That pace would put him ahead of Washington Capitals sniper Alexander Ovechkin’s 65 goals in 2007-08, the apex of single-season goal-scoring marks in the modern era, Travis Yost writes.
By Travis Yost
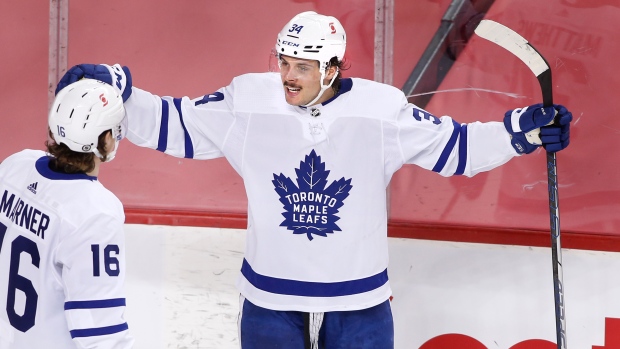
At some point you run out of things to say about the season Toronto Maple Leafs star Auston Matthews is having.
With 38 goals in 47 games, he’s playing at a 66-goal pace over a full regular season. That pace would put him ahead of Washington Capitals sniper Alexander Ovechkin’s 65 goals in 2007-08, the apex of single-season goal-scoring marks in the modern era.
What’s so fascinating about Matthews this season, and in his career more broadly? It’s the consistency with which he scores goals, and his ability to surpass goal-scoring expectations on a routine basis. It has been a common theme with Matthews since he entered the NHL in the 2016-17 season.
Expected goals – which are part and parcel of player-level analysis at this point and quite predictive of future scoring for most players – consistently underrate the danger Matthews poses in the offensive zone. In many ways, Matthews has become the face of the next challenge for hockey statisticians: how to improve the accuracy of expected goal models with high-volume shooters.
In most cases, players float around their expected goal rates after multiple seasons are logged. But high-volume shooters continue to test the limits of public and private models alike. The more these players shoot, the more goals they score – the famous Michael Scott adage is worth recalling here.
But every rule has an exception, or in this case, outliers. Consider the actual versus expected goal rates for Matthews since entering the league, with his shot-on-goal volume overlaid. You’ll notice that his actual scoring rates have persistently dwarfed his expected goal rates; you will also notice that shot-on-goal rates tend to have much more collinearity with his goal scoring.
For his entire career – and on a base of 99th percentile shot volume – Matthews has routinely beaten goal-scoring expectations outlaid by public models. What makes Matthews so interesting is that raw shot-on-goal totals tend to have much higher correlation with his goal-scoring rates than expected goals – expected goals being modelled off shot volume and adjusted for factors that enhance (or diminish) scoring probability, like angles, distances, and shot types.
By and large, this approach works, which pushes Matthews into outlier territory. But it is not lost on me that the other outliers – players like Boston’s David Pastrnak and St. Louis’ Vladimir Tarasenko, as two examples – show similar trends.
Then there is perhaps the best goal scorer ever in Ovechkin. Anyone who has built a model knows it can be broken by the extremes, and Ovechkin certainly qualifies.
Expected goal models have never been able to capture the lethality with which Ovechkin scores goals, and in his case, that trend spans more than a decade:
That is a career-long trend of beating expectations. But not all players with high shooting volumes are proportionally beating goal-scoring expectations. In fact, it’s shooting volume that has become a canary in the coal mine for high levels of variance and lower levels of confidence.
Few players generate more offensive volume than Ottawa’s Brady Tkachuk, who has emerged as a high-end top-six forward with scoring touch – all before turning 21 years old. But if I told you that a first-round pick would enter the league and generate shots at the 99th percentile, with the lion’s share of those shots coming from just outside of the net mouth, you would probably think that player would be near the league lead in scoring.
Obviously that’s not the case for Tkachuk. He’s a great player, but one whose expected goal share always dwarfs his actual scoring:
If you think Tkachuk is alone, think again. Montreal’s Brendan Gallagher and Florida’s Patric Hornqvist show a very similar career trend line.
The one qualitative factor we know differentiates this group from the likes of Matthews and Ovechkin surely encompasses shooting velocity and precision; high-volume shooters can find soft areas in the defensive zone all day long, but if a player can’t find a way to play the angles and beat goaltenders, the goals don’t manifest.
More than anything, I think this points to the limitations of what the hockey stats community can reasonably do with the NHL’s Real-Time Scoring System data – and I say this as someone who thinks the work that has been done off of these data sets is extraordinary, compelling, and has drastically improved our understanding of the sport.
But if someone asked me what’s the singularly most intriguing part of the NHL’s push for puck and player tracking technology, I’d say it’s understanding how goal scorers differentiate from one another and what attributes contribute to goal scoring in the offensive zone.
When we get there, perhaps we will be able to look back on the 2020-21 season and better understand just how Matthews was able to string together such a torrid goal-scoring season.